Putting Data to Work: The Art of Prioritizing Security Measures
With the increasing frequency and scale of cyberattacks, organizations must take proactive measures to secure their systems and data. However, implementing every possible security solution is neither practical nor cost-effective. This is where savvy use of data can guide security priorities.
Contents
Assessing the Threat Landscape
Data is crucial for effectively assessing and monitoring organizations’ evolving threat landscape. By leveraging different data sources, security teams can gain valuable threat intelligence to identify risks, detect attacks, and enhance defenses.
Network and endpoint telemetry provides visibility into systems and user activities to detect anomalies that may indicate threats like malware or insider risks. Log data from firewalls, intrusion detection systems, endpoints, and cloud applications offers rich details for threat hunting to uncover hidden or emerging attacks.
External threat feeds from industry sources give timely warnings about new attack techniques, threat actor groups, vulnerabilities being exploited, and other relevant threats. Genetec helps businesses obtain and analyze this data.
Advanced analytics, machine learning models, and rules engines can process volumes of this data to baseline normal behavior, flag deviations from norms, connect related threat events, and prioritize risks. Dashboards offer security leaders intuitive visualizations of top threats for better decision-making. Risk ratings can highlight critical assets and cyber hygiene issues needing attention, and trend reports track how risks change over time.
By ingesting and correlating data from many internal and external sources, security teams uncover blindspots, validate where controls are working, and continuously fine-tune defenses against a dynamic threat landscape.
Relying on data ensures efforts focus on remediating the most pertinent risks rather than getting distracted by noise or false alarms. Ongoing measurement of key performance indicators for the program also guides process improvements.
Evaluating Security Gaps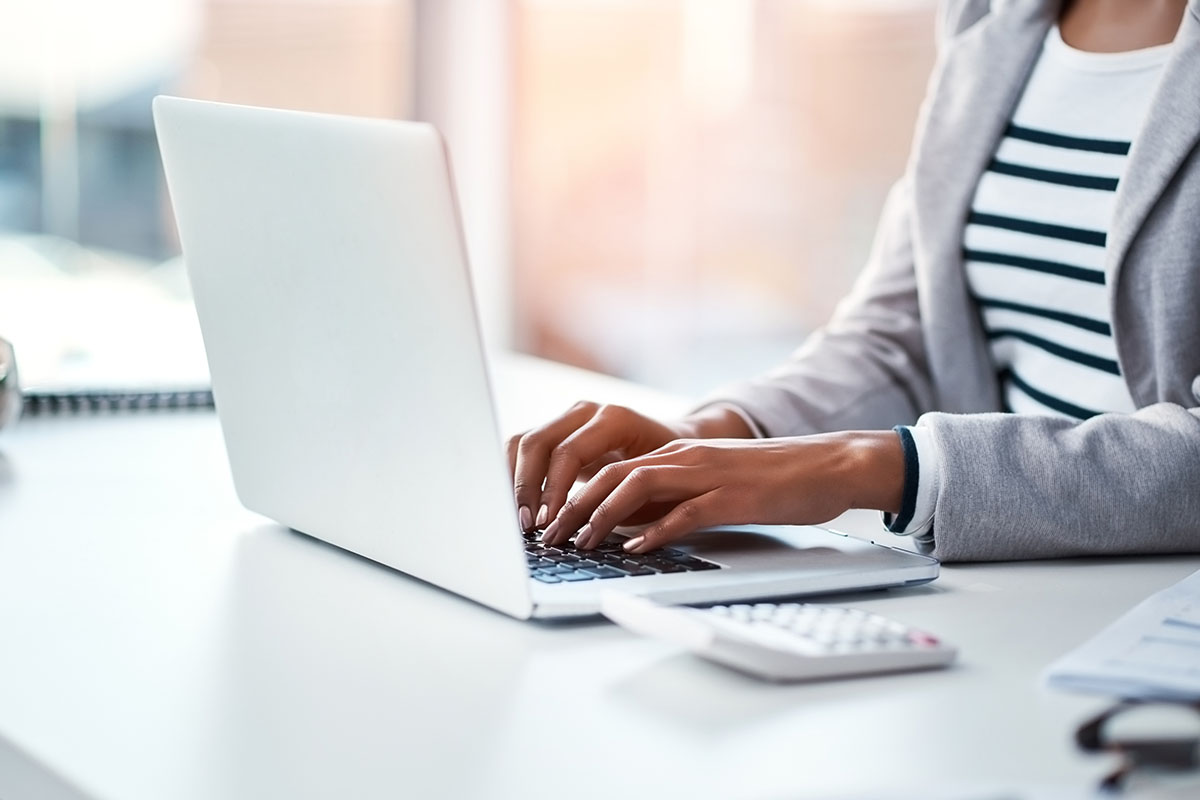
Data analytics help identify and address security gaps within an organization’s systems and processes. By collecting and analyzing various data sources related to user behavior, network traffic, system logs, and more, security teams can gain valuable insights to strengthen overall security. Some key ways data helps evaluate security gaps include:
- Analyzing authentication and access logs to detect anomalous activity like repeated failed login attempts or unauthorized access attempts as security measures. This helps identify gaps like weak passwords, unpatched systems, and others.
- Examining network traffic patterns to uncover communication with suspicious IPs or abnormal volumes of outbound transfers. This enables early detection of malware or insider threats.
- Using behavior analytics tools to create user profiles and identify deviations from normal that could indicate compromised credentials or insiders overstepping bounds.
- Glean insights into policy gaps.
- Correlating audit data across multiple systems to connect the dots on multi-stage attacks. Pinpoint gaps in inter-system segregation or access controls.
- Monitoring support tickets or bug tracking systems to identify commonly reported issues. Uncover gaps in security awareness or technical configurations.
With data providing various breadcrumbs on user actions and asset security, analysis can reveal blindspots and weaknesses in security infrastructure, ranging from human errors to technical flaws. A continuous feedback loop of monitoring data, identifying gaps, and strengthening controls are key to maintaining robust cyber defense with a proactive mindset.
Calculating Risk Scenarios
Data analysis is crucial in calculating and planning for risk scenarios across many industries. Whether in finance, insurance, business operations, or disaster response planning, having detailed data enables more accurate quantitative risk assessment. This data might include historical trends, real-time monitoring, predictive modeling, or simulations based on various what-if situations.
For example, an insurance company can analyze vast amounts of claims data from many years to detect patterns and frequencies of events like floods, fires, or car accidents in different geographical areas. This allows them to quantify these recurring events’ probability and impact costs. They can adjust risk premiums accordingly and ensure sufficient reserves or reinsurance to remain solvent even in a historically unprecedented worst-case disaster scenario.
Similarly, financial institutions use data analysis ranging from macroeconomic trends to individual consumer behavior patterns detected through AI to build risk models that simulate the impact of various downturns or crises.
The models provide actionable information, such as where vulnerabilities exist in their lending portfolios if a certain percentage of borrowers defaulted under a recession over a specific timeframe. Institutions can then make data-driven decisions to mitigate these risks proactively.
The availability of big data and modern analytical tools allows very robust statistical risk measurements and simulations to be performed across sectors. However, data quality and choosing appropriate models aligned to purpose are vital to quantifying the likelihoods and impacts in a realistic and actionable way.
Implementing Safeguards Strategically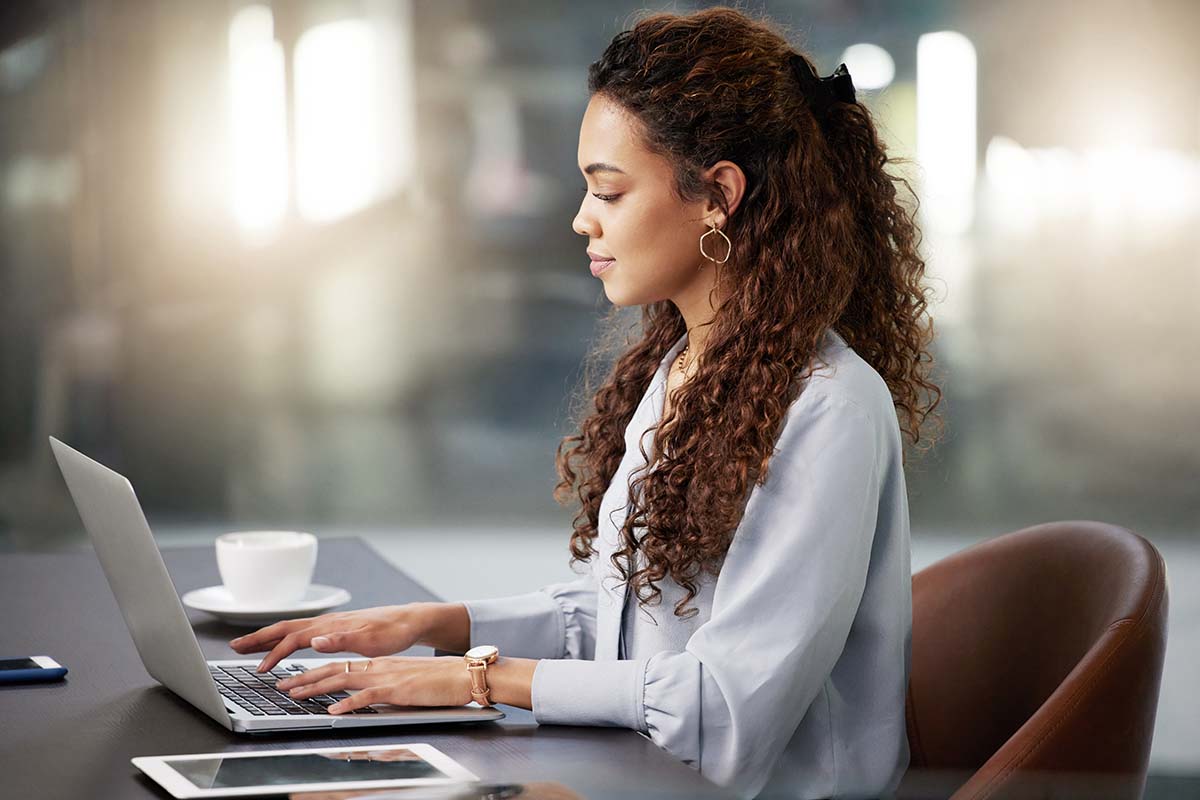
Implementing effective safeguards requires a strategic, data-driven approach. Organizations can leverage data analytics to identify their most critical assets, vulnerabilities, and potential threats. For example, customer data, intellectual property, and core systems like accounting or inventory may emerge as top priorities to protect based on business impact assessments.
With critical areas highlighted, leaders can then utilize risk assessments to reveal specific weaknesses that could be exploited. These may range from legacy software lacking updates to inadequate access controls around sensitive information. Granular data around past incidents and emerging attack trends from both internal teams and external providers gives helpful context for where attention is warranted.
With these insights, executives can prioritize and strategically layer safety measures according to potential risks and resources. Quick wins like updating vulnerable software or implementing multi-factor authentication may be prudent first steps. Longer-term safeguards like modernizing infrastructure, automating monitoring capabilities, and training employees can then be systematically followed based on budget and roadmaps set using the risk data and security measures.
Ongoing success relies on continuously gathering updated data through audits and testing. Emerging trends around new attack vectors and compliance demands should feed back into the strategic planning process. Following the data insights, directing resources toward identified weak spots, and modernizing defenses over time provide an adaptive approach for implementing layered, strategic safeguards over time.
Security Measures: Repeating the Cycle
Risk assessments and implementation plans cannot remain static in today’s ever-evolving threat environment. Regular repetition of the data analytics cycle is essential to keep pace. For example, every 6 months, gathering the latest threat intelligence, reevaluating defenses, and recalculating priorities allows security to stay dynamic.
Although perfect security is unattainable, optimizing defenses by zeroing in on the most likely and most damaging attack vectors can make organizations resilient. The foundation is bathing decisions in data, from threat assessments to risk analysis and tracking risk reduction over time. Turning metrics into meaningful actions is how security leaders can do more with less.